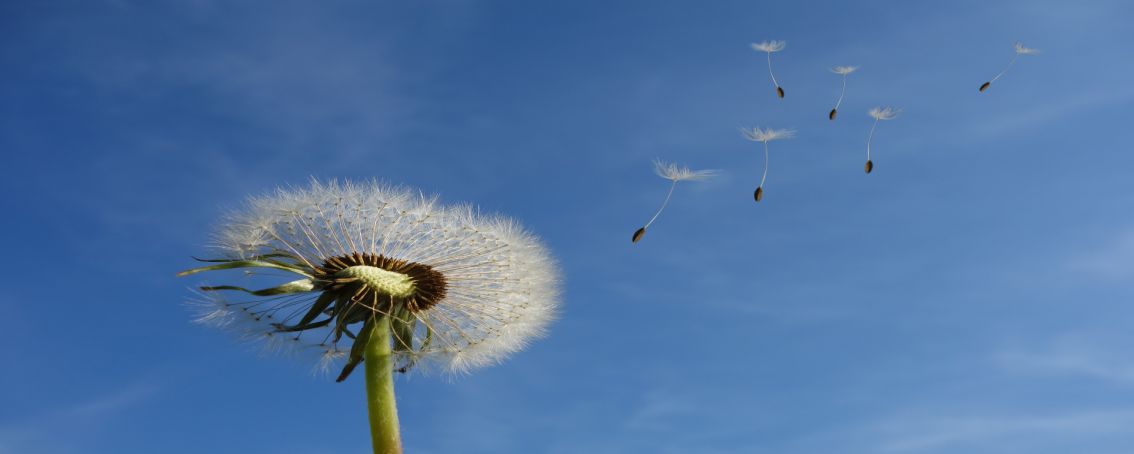
This article discusses how tokenization may reduce false declines with credit card transactions that could negatively impact merchants.
The Problems that False Declines Cause Merchants
In the card processing industry, a false decline occurs when a customer, who in good faith, and within their spending limit, sees his transaction rejected. While this is embarrassing for customers, it can cause problems for merchants.
A high volume of credit card declines can have a very negative impact on merchants. This may include penalties from the payment network. These penalties could even gradually increase if the high denial ratio remains unsolved over a long period of time.
False declines are not a small problem for merchants, Sometimes it may even have a more serious impact than the fraud itself. On average, around $8.6 billion CNP transactions per year are declined by U.S merchants but the fraud it prevents is only $6.5 billion in E-commerce (CNP transactions). This means that for U.S. E-commerce, the false decline volume is about $2.1 billion per year. However, the real cost of false declines goes far beyond that figure.
False declines are often an “invisible” problem for the merchants as they may not be fully aware of how many of their customers’ cards were wrongly declined. This is an error for tracking anti-fraud performance for chargeback rates only that turns a blind eye on the false positive rate, which is often much harder to track.
Usual Reasons for False Declines
The reasons for false declines are usually complex. The customer is also not informed about why the card has been declined. In a card-present transaction, he might receive a “Do Not Honor” message. However, while in a card-not-present transaction, that customer will almost always be presented with a generic message like “transaction refused” or “the card cannot be charged”. [1]
Under the hood, the reasons may be different:
- The Anti-Fraud system of the merchant wrongly refused the transaction;
- The Anti-Fraud system of the acquirer wrongly refused the transaction (for instance, false positive in AVS- Address Verification)
- In the transaction chain, some risk management systems were tuned in a “too cautious” way;
- The issuer bank may suspect fraudulent use of the card.
An anti-fraud system could be using “behavioral” analysis and “heuristically” detect a “strange” and “unusual” transaction. For example, consider that Mr. Jones has been only buying cheap $5 DVDs for 5 years and all the sudden he wishes to buy a brand new, $2,000 MacBook online. While Mr. Jones has the funds in his bank account and is within his rightful spending limits, the anti-fraud system could consider the transaction as very unusual and block it.
Anti-Fraud systems often use processes, such as Bayesian Classifiers, a type of “artificial intelligence.” These systems “learn” to predict what will be a fraudulent transaction by looking at a set of parameters (name, issuer bank, IP address, country of residence, etc.). They then return a score that will be added to other tests, and the end result might block a legitimate transaction.
The same happens with your email’s SPAM folder. Obviously, there is a lot of real spam in it, but often valid emails end up in the folder, too. This means that the Bayesian prediction system also has its own limits.
This problem is well-known to engineers involved in access control (for instance, biometric access control). With anti-fraud systems, false positives (good people are refused access) and false negatives (bad people are granted access) work in inverse proportions. The more “relaxed” you are with acceptance, the more you will wrongly allow the “bad” people to enter. The more you are “harsh” with acceptance, the more you wrongly prevent the “good” people from entering.
A good scenario for invoking a false decline is to buy from an online Swiss merchant with a French credit card while using a German IP address. Most victims of false declines are usually young, mobile, often involved in tech, and buy online relatively frequently.
Unfortunately, in the payment card industry, reducing false decline is not a small problem.
Why False Decline in CNP Can Be Worse Than Fraud Itself
In a case of a successful fraudulent transaction (false negative), the losses are not equal to the actual volume of the fraudulent transaction. The losses are equal to the gross cost of the product plus the shipping fees. That cost can be around 100% (jewelry, gold) or 0%( digital goods) of the price in the shop. For example, in the case of clothing, this cost will be the manufacturing cost, which can be very very low, around 5% of the product price (e.g. sports shoes).
However, in the case of false positives, the loss may be much more damaging.
In E-commerce there is no human operator to guide the customer. In most cases, the customer may not try again to buy the product and will look for a competitor. The customer will keep a painful memory of the episode and negative image of the merchant will remain as well. This is a customer who is mostly lost, who could have been a loyal customer, and who could have referred the shop to other potential customers.
In the case of ultra-competitive merchants, the impact of false-positive declines can be significant. Using 3D secure protocols could also lead to false-positives, and often they are not sufficient to prevent fraud.
Surprisingly, tokenization can be a solution to reduce false declines.
How Does Tokenization Reduce False Declines?
Reduces Risky Elements Passed to the Merchant
A merchant using a tokenization solution will not ask for credit card numbers but instead, a token after initial enrollment. The customer inputs their username and authentication credentials. The merchant fetches the token and passes it to the card acquirer in lieu of the real PAN. In situations like this, the anti-fraud controls become a little “friendlier” because there are fewer risky elements in the transaction.
Allows for Building Better Card-on-File Systems
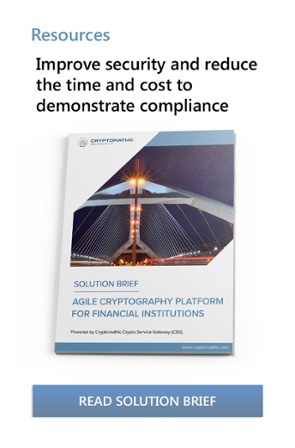
As a side effect of tokenization, merchants will be able to build improved card-on-file systems where tokens are stored in a riskless way and outside the scope of PCI compliance. In such situations, merchants will provide their customers with a 1-click or 1-touch payment button.
There will be no reason to trigger their anti-fraud systems against a well-known recurring customer with a card which is known. Instead, the anti-fraud controls will be at the level of the login and authentication.
Such a simplified checkout will reduce false declines because even if card risk management can flag the transactions at an acquirer or issuer level, it will never be flagged by the merchant (at least after an initial period). Storing tokenized cards reduces the risk of data mismatch or outdated data which could flag the transaction.
Obtaining Risk Information Within De-Tokenization
The most effective way tokenization could reduce false declines is by passing risk-associated data to the acquirer during de-tokenization.
Note that the Visa Token Service (VTS) and the Mastercard Digital Enablement Service (MDES) are integrated with the latest 3D secure 2 protocol (3DS2). Merchants that were part of the network tokenization pilots mentioned that they experimented a false decline reduction between 5-8%.
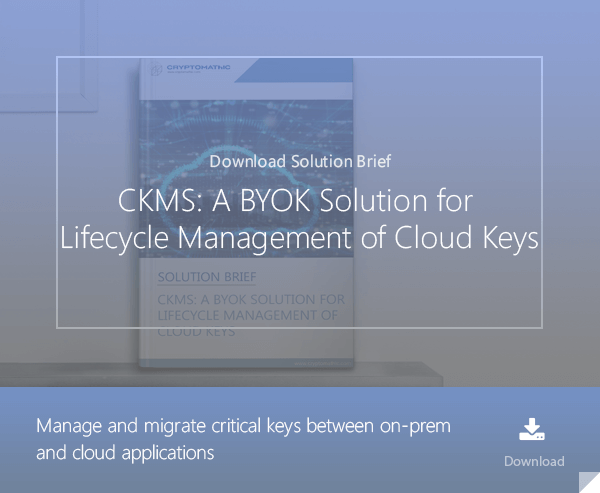
References, Footnotes and Further Reading
- [1] This is of course done on purpose so that criminals could not guess how the anti-fraud system works and adjust their “experiments” because the error message is a generic one.
- More articles on tokenization (2018 - today), by Martin Rupp, Dawn M. Turner, and more.
- More articles on Crypto Service Gateway (2018 - today), by Chris Allen, Jo Lintzen, Terry Allen, Rob Stubbs, Stefan Hansen, Martin Rupp, and more.
- Tokenization Product Security Guidelines (April 2015), by the PCI Security Standards Council, April 2015
- Payment services (PSD2) - Directive (EU) 2015/2366 (2015) by the European Commission